Nano-Robotics
Circuits Heading link
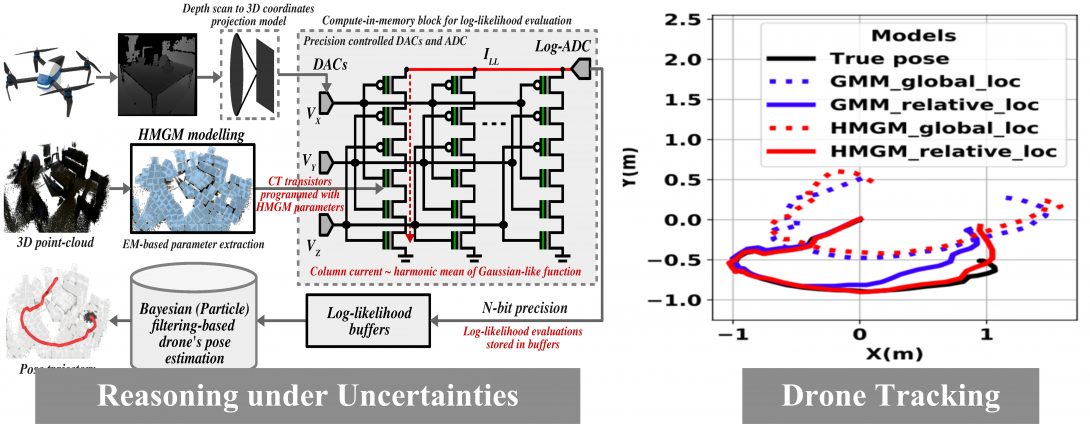
Autonomous insect-scale drones open intriguing application prospects. In an agriculture field, insect-scale drones can locate infected plants to prevent disease spread. In an industrial plant, they can alert about gas leaks by navigating through the constricted pipelines. During earthquakes, the drones can assist in search and rescue by navigating through building debris. Meanwhile, since an insect-scale drone can only carry a small battery’s payload, minimizing power dissipation for on-board processing becomes quite critical. An insect-scale drone must also operate with only a few lightweight sensors since each sensor demands more power and increases the vehicle’s size and weight. While the cloud can be leveraged to circumvent the above on-board processing, reliable continuous connectivity to the cloud cannot be assumed in many applications.
For ultra-low-power autonomy in insect-scale drones, we came up with a novel representation of 3D maps using a harmonic mean of the Gaussian-like” mixture (HMGM) model. We showed that, unlike a traditional map model, HMGM-based models could be computed with much higher efficiency and enable a similar navigational accuracy. Specifically, I discussed that HMGM-based map models could be easily emulated by an array of multi-input floating gate CMOS inverters. Our approach led to 450x lower energy than the traditional for drone localization (i.e., tracking of drone’s position and orientation in 3D space). Also, using the procedure, not only the prediction itself, but the prediction confidence was also measurable to handle the flying domain’s uncertainties. This research is being supported by Discovery Partner Institute.